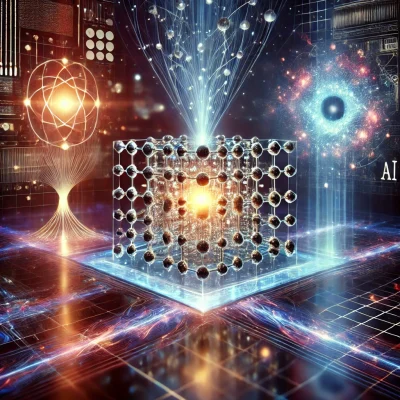
Quantum Computing: Revolutionizing Data Science and AI
Introduction The exponential growth of data and the increasing complexity of artificial intelligence (AI) models demand computing power beyond the capabilities of classical systems. Enter quantum computing, a transformative technology that has the potential to revolutionize data science and AI. By leveraging quantum mechanics principles, quantum computers promise breakthroughs in processing speed, optimization, and problem-solving that were previously unimaginable.
Key Concepts in Quantum Computing
- Qubits vs. Classical Bits: While classical bits are binary, qubits can exist in superposition, representing multiple states simultaneously.
- Entanglement: Quantum entanglement allows qubits to be interconnected, enabling faster and more efficient computations.
- Quantum Gate Operations: Unlike classical gates, quantum gates manipulate qubits using the principles of quantum mechanics, introducing unique problem-solving approaches.
Applications of Quantum Computing in Data Science and AI
- Optimization Problems: Quantum algorithms, like QAOA (Quantum Approximate Optimization Algorithm), can solve complex optimization tasks faster than classical counterparts, aiding in tasks like supply chain management and portfolio optimization.
- Machine Learning Acceleration: Quantum computing can speed up training and inference in AI models, particularly in areas like natural language processing and computer vision.
- Data Clustering and Pattern Recognition: Quantum-enhanced algorithms, such as quantum k-means, promise more efficient and accurate clustering in large datasets.
- Cryptography and Security: Quantum computing also presents challenges and opportunities in data encryption, enabling new secure protocols like quantum key distribution.
Challenges and Limitations
While quantum computing holds immense promise, it faces significant hurdles:
- Hardware Scalability: Building and maintaining stable quantum hardware is still a significant challenge.
- Algorithm Development: While promising, quantum algorithms for AI and data science are still in their infancy.
- High Costs: Quantum computing resources are expensive and currently accessible to only a few organizations.
Future Outlook
Despite these challenges, quantum computing is advancing rapidly. Collaborations between tech giants, research institutions, and startups are accelerating progress. Over the next decade, we can expect significant strides in integrating quantum computing into mainstream data science and AI workflows.
Conclusion
Quantum computing is not just a futuristic concept; it is gradually becoming a practical tool that could redefine how we approach data science and AI. As the technology matures, the integration of quantum solutions will unlock unprecedented capabilities, pushing the boundaries of innovation and discovery.